![]() | Hello, Ethereum subreddit community! As someone who's been in engineering leadership roles, I've always had this question bobbing around in my mind: how can we really measure an engineer's contribution to a project in a clear and fair way? My longstanding participation in the crypto space has only made this question loom larger, especially with the whole idea of trustless, permissionless environments. So, I've put together a little essay on this. I hope you find it interesting and that it can pop up some ideas on you too. IntroI've been particularly interested in a scenario where one could measure an individual contributor's engagement and contributions based solely on GitHub and the artifacts that this individual generates through their workflow. This includes actions such as code commits, pull requests, and code reviews particularly in a permissionless and decentralized environment. In this setting, traditional management tools like Jira and commonly used DORA metrics including Cycle Time and Lead Time are often unavailable or impractical to implement. Analyzing the engineering metrics generated directly from the codebase allows us to measure an engineer's contributions and engagement in a clear, straightforward manner. Moreover, this approach is well-suited for open-source projects, where interactions are primarily centered around the codebase and there's limited scope for other forms of engagement. No doubt many of you, like myself, have participated in organizations that employ tools like Jira to manage workflows and measure individual contributions, often using metrics such as story points. I can recall many instances throughout my career where I felt frustrated by such systems. In large organizations, it can be particularly challenging to gain visibility for the hard work of individual contributors. It's not uncommon for middle management to be introduced to identify top performers, a process that can often devolve into a game of social capital. This can lead to inefficient workflows and, in some cases, a politicized environment that detracts from the organization's main goals and the individual's experience. Imagine this now: What if we could come up with a super precise way to measure who's doing what in these projects? It could be a game-changer. We might finally crack the code on how to make money from open source work. Communities could start selling products or services, and here's the best part - the money made could be shared out among everyone involved, based on how much they've contributed. This could totally revolutionize how we work together and create value in the open source community. Clearly, a venture is more than just the sum of its engineering contributions. It's a complex ecosystem that includes multiple roles, such as designers, project managers, and marketers, each contributing to the overall success in unique ways. It's crucial to understand this holistic view of a venture. That being said, there is a subset of problems that companies are currently solving which could realistically be managed entirely by self-coordinated engineering teams. A prime example of this is open source software. While I believe that one day we will be able to deterministically measure all types of contributions, whether from a designer, project manager or marketer, the reality is that we are not quite there yet. However, measuring engineering contributions is a not-so-low hanging fruit that we can start with. This is not to diminish the importance of other roles, but rather to acknowledge the practicality and immediate feasibility of quantifying engineering impact. AI x CryptoWhile much of the tech world is buzzing about the potential of AI to boost engineering productivity and enhance developer tools, there is an equally important aspect that is often overlooked: the power of AI to measure that productivity and those contributions. This is a side of the coin that, unfortunately, tends to be dismissed or even frowned upon. Many people perceive such applications of AI as oppressive or a means to weaponize data at the expense of worker exploration. However, IMHO, this perspective fails to grasp the transformative potential of these technologies. Rather than being a tool for oppression, AI can serve as an enabler for true collaboration within a permissionless paradigm. It can unlock an entirely new way of working, lowering barriers to access capital and fostering collaboration among individuals who share the same ideas and are working towards a shared goal. By quantifying contributions, AI can provide an objective basis for recognizing and rewarding effort, thereby promoting a more equitable and inclusive work environment. While the advent of Large Language Models (LLMs) has brought about significant advancements in AI, it's essential to recognize their limitations. LLMs are not well-suited for deterministic scenarios or strict logic, as they cannot reliably form a chain of logical conclusions. Their capacity to construct and follow complex logical sequences is limited, which can pose challenges in tasks requiring rigorous logical reasoning. However, where LLMs truly shine is in evaluating subjective matters such as code quality. Especially with the recent explosion of context tokens available on consumer-grade tools. We'll be delving deeper into the topic of Large Language Models (LLMs) later on, but it's worth noting here that they can offer significant enhancements to existing research methodologies aimed at capturing the impact of contributions. LLMs bring in a non-deterministic and subjective aspect that is usually only created through human interaction, adding a new layer of richness to the analysis that goes beyond traditional programmatic approaches. Another significant advantage of Large Language Models (LLMs) is their potential to help with abuse prevention, an area in which previous algorithms fell short. LLMs can be instrumental in detecting contributors who are merely "farming" points and not providing any meaningful contributions. This increased level of scrutiny can ensure that points are awarded based on genuine contributions, enhancing the fairness and integrity of the system. Micro-DAOs, Collaborative SaaS.For some of you, this concept of collaboration within a permissionless environment might ring a bell, especially when it comes to DAOs. I must admit, I have a love-hate relationship with DAOs. On one hand, the concept is absolutely phenomenal. Yet, on the other hand, the execution often leaves much to be desired. DAOs are a complex topic, and while I won't delve too deeply into it here, one thing is clear: the barrier of entry for newcomers to the ecosystem is high, particularly when it comes to creating DAOs. The considerations are numerous: from determining voting mechanisms and proposal acceptance criteria to designing the governance structure and the number of initial participants. Starting a new venture as a DAO often requires a significant upfront investment of time and resources simply to establish the functional framework. This complexity and resource intensity can deter many from taking the plunge into the DAO world. Over recent years, there has been a remarkable advancement in the tools available for DAOs, making the process of creating one considerably less daunting than it was just four years ago. However, if you wish to establish something truly unique that mirrors your organization or community's values, you still need to develop your own smart contract, and it better be audited⦠Nowadays, tools like Coordinape and Karma allow for a more streamlined management of DAO operations. However, the complexity of these operations still poses a significant challenge, especially for newcomers stepping into the web3 world. To some, it may seem an insurmountable task. The most effective approach, in this case, is to first participate in a DAO, to understand its complexities and intricacies. This will allow you to build a community around your idea and understand the parameters involved. Only after this you can consider creating a DAO that could generate value for your community or for yourself. The duality of DAOs has always intrigued me. On one hand, their permissionless nature and purpose are aimed at lowering barriers to collaborative work, fostering a space for people to unite towards a common goal. However, in reality, the complexities inherent in DAOs often result in the opposite effect. The high barriers to entry, primarily due to their intricate nature, can deter many potential collaborators. What we need is a starting point that is accessible and requires little resources to explore. It must be capable of organic growth, both in terms of size and complexity, but only when necessary. We need a way for individuals to collaborate in a manner akin to their current work practices. If such a system could generate value for its participants, it should also possess the flexibility to adapt and transform according to the evolving phases of the organization, while providing financial tools to sell products and services from the beginning. The status quoI hope by now I've provided a compelling case for why this is an important problem to tackle, and how various types of organizations could stand to reap significant benefits from such a metric. Whether it's open-source projects, traditional corporations, or DAOs, there is a broad spectrum of entities that could find immense value in having a quantifiable measure of individual engineering impact. But now, we're getting to the most interesting part - how exactly can we achieve this? Now, we are witnessing the emergence of initiatives like Open Source Observer, which are designed to provide meaningful data around the impact of projects. Pioneers such as Optimism RPGF and Gitcoin have paved the way to raise awareness about such impact metrics in the realm of public goods projects, pushing this matter into the mainstream conversation. While impact metrics for projects within an ecosystem, or within web3, are receiving increasing attention, we still need to highlight the immense value that impact metrics for individuals can bring to web3 and to society in general. The potential use cases are vast and we are just at the beginning of understanding what we can achieve with it. This peculiar obsession of mine has led me down several different paths of research. Yet, one common factor amongst all the methods I've explored is their lack of real-world experimentation and productization. While the majority of the material and solutions are tucked away in the academic world, a few open-source projects are "almost" usable and provide a good framework to follow. However, at their core, most of these methods use the same raw metrics as a starting point:
These metrics provide a fairly accurate picture of an engineer's engagement and impact on a project. On the other hand, metrics like commits or deployments might not be as straightforward or meaningful in this context. For instance, the number of commits a developer makes doesn't necessarily correlate with the quality or importance of their contributions. Similarly, deployments are often dependent on other factors beyond an individual engineer's control. Therefore, our focus should be on the metrics that most accurately and fairly represent an engineer's contributions to a project. Here's where things start to get tricky. How do you actually measure that data? Do you simply sum up the number of pull requests of each engineer and compare them? But what is more valuable? An issue or a pull request review? A real experiment I stumbled upon a project called SourceCred in 2020, and they have created a great methodology to measure individual impact. There are a couple of academic papers that used SourceCred to analyze contributions, and similar approaches to the same problem, and although not popularized, it made big waves among collaboration enthusiasts when it was alive Back in its early days, SourceCred received funding from Protocol Labs and was led by two Google engineers who had previously worked on PageRank and TensorFlow respectively. For those unfamiliar with PageRank, it's Google's proprietary algorithm used to measure the relevance of a website in relation to others based on a specific search query. The key element here is the concept of "relevance in comparison to others". This is the core principle that SourceCred adopted and applied to contributions. It constructed a graph where both contributions and contributors are represented as nodes. These nodes are interconnected with edges, the weights of which are determined based on the relevance of both the contributors and the contributions themselves. There are several intriguing aspects of how this algorithm calculates the weights of these edges. Perhaps one of the most fascinating approaches is the inherent retroactivity of contributions. If a specific artifact references an old issue at a later date, the relevance of that issue increases based on the relevance of the artifact that referenced it. This retroactive approach ensures that contributions are valued not just in the moment they're made, but over the course of the project's lifespan, acknowledging the long-term impact of contributions. However, it's important to note that SourceCred is not without its flaws. Notably, the system is susceptible to Sybil attacks, where an entity illegitimately inflates its influence by creating multiple identities. Additionally, SourceCred currently lacks the ability to account for qualitative metrics. While this might seem fair at a superficial level, it's clear that this approach falls short in capturing the nuance of real-world scenarios. This is where the power of AI comes into play. With the recent explosion of capabilities in consumer-accessible Large Language Models (LLMs) and the increase in available context tokens, such as the recent release of Grok, we can successfully add qualitative heuristics to these contributions, taking into account the entire context of the project, and prevent or at least decrease the abuse potential. However, it's important to note that there is a limit to how much context an LLM can handle effectively. For instance, it is currently beyond the scope of any LLM to handle an enormous codebase like that of Google in its entirety... at least for now. Unfortunately, SourceCred's journey was not all smooth sailing. The project stopped receiving funding from Protocol Labs and consequently, it faded away. An in-depth ethnography by Ellie Rennie provides an excellent account of what transpired during this period. In an ambitious leap, the SourceCred community sought to measure contributions from various platforms, including Discord, Discourse, and others. However, this approach proved too expansive and ultimately opened the door to abuse and exploitation. The algorithm was simply not equipped to handle contribution patterns from these diverse sources. This experience strengthens my belief that we should first strive to solve a small subset of the problem very well through experimentation before thinking about measuring other types of collaborations that are much less deterministic than code. Despite the cessation of funding, a small community still surrounds SourceCred. However, active development contributions to the project are no longer ongoing. As a result, the SourceCred algorithm has become increasingly unfriendly for newcomers. The setup is complex, and there's a substantial journey involved in addressing compatibility issues and filling gaps in the documentation. Unfortunately, it's not an easy task to set it up and measure any contribution type, especially today as the project becomes more deprecated, given it's in Javascript instead of the more modern Typescript. ConclusionThe possibilities here are endless, and there are no right or wrong answers. This is something we can only achieve through experimentation, constant iteration, and insightful feedback from people in a permissionless environment. I've created an open-source tool to help people achieve just that: https://armitage.xyz We're using SourceCred as a base and plan on expanding it with qualitative AI heuristics. Currently, we are in the process of iterating and exploring what those quality metrics might be. And hopefully receive funding to also modernize sourceCred to Typescript. Although we are simply an MVP, the tool is already beneficial to some teams who are compensating their contributors using Armitage scores. We welcome contributions, and we are ourselves a meta-experiment, measuring contributions within our project for future rewards. [link] [comments] |
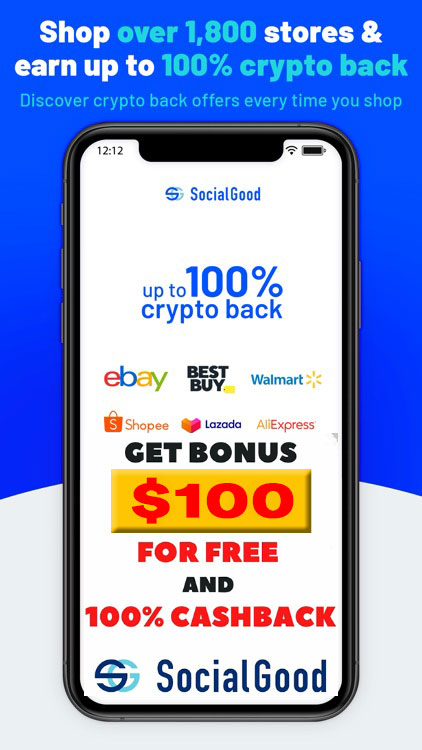
You can get bonuses upto $100 FREE BONUS when you:
π° Install these recommended apps:
π² SocialGood - 100% Crypto Back on Everyday Shopping
π² xPortal - The DeFi For The Next Billion
π² CryptoTab Browser - Lightweight, fast, and ready to mine!
π° Register on these recommended exchanges:
π‘ Binanceπ‘ Bitfinexπ‘ Bitmartπ‘ Bittrexπ‘ Bitget
π‘ CoinExπ‘ Crypto.comπ‘ Gate.ioπ‘ Huobiπ‘ Kucoin.
Comments